The area of quantum machine learning (QML) has received tremendous attention as researchers discover the potential synergy among quantum computing and device-learning algorithms. This rising discipline combines the standards of quantum mechanics with conventional devices, gaining knowledge of techniques to remedy complex problems more successfully.
Introduction
With its capacity to handle records concurrently and make use of quantum phenomena like superposition and entanglement, quantum computing offers promising opportunities for boosting various components of system learning. By utilizing those specific residences, quantum machine learning targets conquering the constraints confronted via classical computer systems when handling huge-scale datasets and computationally intensive responsibilities.
Machine learning algorithms shape the backbone of quantum machine learning, allowing researchers to educate models on quantum computer systems using quantum facts. These algorithms are tailored or designed mainly for quantum systems, utilizing their built-in parallelism and modern methods like dimension-based computation and significance encoding.
We will delve into the fundamental ideas at the back of quantum machine learning and discover the way it differs from classical processes. We will study key components, which include variationally circuits, qubit encodings, and hybrid fashions combining classical and quantum components, and examine their packages in different domains. By knowing the basics of quantum machine learning, readers will gain insight into this thrilling discipline at the intersection of quantum computing and gadgets.
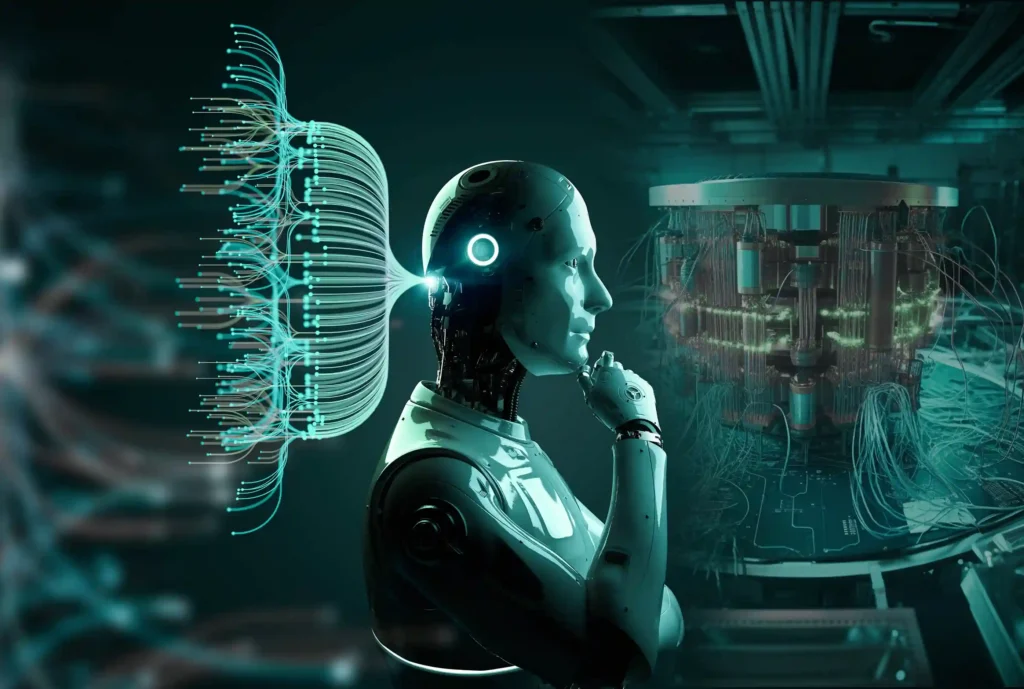
With its capacity to handle records concurrently and make use of quantum phenomena like superposition and entanglement, quantum computing offers promising opportunities for boosting various components of system learning. By utilizing those specific residences, quantum machine learning targets conquering the constraints confronted via classical computer systems when handling huge-scale datasets and computationally intensive responsibilities.
With its capacity to handle records concurrently and make use of quantum phenomena like superposition and entanglement, quantum computing offers promising opportunities for boosting various components of system learning. By utilizing those specific residences, quantum machine learning targets conquering the constraints confronted via classical computer systems when handling huge-scale datasets and computationally intensive responsibilities.
The Advantages of Quantum Machine Learning Over Classical Machine Learning
Quantum devices gaining knowledge hold first-rate promise in remodeling the sphere of synthetic intelligence. By applying the energy of quantum mechanics, it offers numerous benefits over classical gadget-studying strategies.
The most important advantage of quantum devices is their more desirable computational electricity. Quantum computers can carry out complex calculations and process significant quantities of facts simultaneously, resulting in an exponential speedup compared to classical computer systems. Faster processing speeds and the effective execution of increasingly complicated and sophisticated algorithms are made viable by this.
Quantum machine learning algorithms have the capacity to attain improved accuracy and performance. Quantum systems can push quantum entanglement and superposition to discover a couple of possibilities concurrently, permitting a more complete analysis of statistical styles. Better forecasting and selection-making skills may also result from this.
Another advantage is that quantum machine learning knowledge of algorithms offer stability in opposition to noise and mistakes. Quantum mistake correction strategies can mitigate the results of carelessness, which is a prime task in quantum computing. This guarantees that the computations will continue to be reliable even in the presence of external disturbances.
Quantum systems gain knowledge and have the capability for innovations in solving complicated optimization issues. Many actual-international challenges involve locating the most advantageous answers inside massive answer spaces, which includes portfolio optimization or logistics planning. Promising methods for fixing those optimization issues more correctly are supplied by quantum algorithms consisting of variationally algorithms and quantum annealing.
Quantum machine learning gives full-size advantages over classical device mastering strategies, such as more suitable computational energy, quicker processing speeds, stepped-forward accuracy and performance, in addition to sturdiness towards noise and mistakes. As research progresses in this field, we can assume further advancements so we can shape the destiny of artificial intelligence applications across numerous industries.
Applications of Quantum Machine Learning in Various Industries
Quantum machine learning, the fusion of quantum computing and system getting to know, has emerged as a promising subject with programs throughout various industries. In this segment, we can discover some of the key applications of quantum machine learning in one-of-a-kind sectors.
One area in which quantum devices getting to know themselves suggests splendid capacity is drug discovery and improvement. The complex nature of molecular interactions and the substantial search area for capability drug candidates make it an ideal use case for quantum algorithms. Researchers can discover new compounds more quickly and more appropriately predict their efficacy by making use of the power of quantum computing.
Financial modelling and prediction are any other area where quantum machine learning can have a tremendous impact. The capability to process large amounts of data quickly and effectively permits more accurate forecasting models for inventory charges, market trends, and danger assessment. This can aid economic establishments in making knowledgeable selections and enhancing funding techniques.
Optimization troubles in logistics and supply chain management are widely tough due to their complexity. Quantum device mastering algorithms have verified their capacity to fix those optimization troubles more effectively than classical methods. This can result in improved route planning, inventory management, and normal operational performance inside supply chains.
It’s important to observe that while these programs maintain promise, quantum machine learning is still in its early stages of development. As generation advances in addition, we are able to assume that even more modern use cases emerge across industries.
The integration of quantum computing with system mastering opens up new possibilities for solving complex problems in diverse sectors, including drug discovery, finance, logistics, and supply chain control. As research progresses and technology evolves, we are able to anticipate interesting improvements in those fields driven by the strength of quantum machines and their knowledge of algorithms.
The Challenges and Limitations of Implementing Quantum Machine Learning Algorithms
Implementing quantum machine learning algorithms presents several demanding situations and boundaries that need to be addressed for successful software. One sizeable project is the presence of quantum noise and inappropriate results. The underlying weak spot of qubits, the fundamental units of quantum computing, gives an upward push to those effects. Calculations can include mistakes due to quantum noise and decoherence, which could produce erroneous effects.
Another hindrance is the contemporary country of the Qubit era. Qubits are at risk of errors and feature a confined lifespan before decoherence occurs. This limits the complexity and scale of computations that may be done with the use of current quantum computer systems.
To deal with these challenges, researchers are actively exploring blunder correction strategies for qubits. These strategies aim to mitigate errors by encoding facts time and again throughout multiple qubits and enforcing mistake detection and correction protocols.
A primary issue lies in the loss of large-scale quantum computers available for sensible use. Building such structures with a wide variety of qubits remains a strong technological project.
Despite those demanding situations, progress is being made in growing more strong qubits, improving error correction techniques, and scaling up quantum computing skills. As these improvements continue, we are able to count on them to overcome many obstacles related to enforcing quantum gadgets and gaining knowledge of algorithms.
Promising Research Areas in Quantum Machine Learning for Future Development
Quantum machine learning is a rising area that combines the ideas of quantum computing with system learning algorithms. It holds exquisite promise for future development and has the potential to transform various industries.
One promising study place in quantum gadget getting to know is the exploration of hybrid classical-quantum techniques. These strategies intend to use the benefits of both classical and quantum computing to solve complicated issues more effectively. By combining classical system mastering techniques with quantum algorithms, researchers can beautify the abilities of current models and enhance their performance.
Another exciting avenue of study is the development of quantum neural networks. These networks make use of quantum standards, inclusive of superposition and entanglement, to systematize and examine facts. Quantum neural networks have proven promise in fixing complex pattern-popularity tasks and have the capacity to outperform classical neural networks in certain applications.
Researchers are actively investigating the use of quantum guide vector machines (SVM) in gadget study obligations. SVMs are extensively used for type and backwardness troubles in traditional machine learning. By utilizing the electricity of quantum computing, it is possible to increase the efficiency of algorithms that could manage large datasets with stepped-forward accuracy.
There are ongoing studies on developing quantum reinforcement study algorithms. Training an agent to make choices primarily based on input from its surroundings is referred to as reinforcement learning. Quantum reinforcement mastering algorithms intend to exploit the particular houses of quantum structures, including superposition and interference, to decorate decision-making techniques.
Promising research areas in quantum machine learning include hybrid classical-quantum methods, quantum neural networks, quantum aid vector machines (SVM), and quantum reinforcement learning algorithms. Continued improvements in these areas preserve huge capacity for destiny tendencies in diverse industries that depend on gadget-getting-to know technologies.
FAQ
Quantum machine learning is an interdisciplinary subject that combines standards of quantum computing and classical system mastering to increase the number of new algorithms and fashions. It uses the unique properties of quantum systems to resolve complicated troubles more effectively than classical computers in a few cases.
Quantum computing differs from classical computing in its fundamental computing gadgets, called qubits (quantum bits), which could exist in a couple of states simultaneously because of superposition and may be entangled with each other. This enables quantum computers to carry out positive computations, including searching through massive databases or factoring big numbers, lots faster than classical computer systems.
Quantum machine learning makes use of numerous quantum algorithms, with one of the most well-known examples being Grover’s set of rules for looking at unsorted databases. Others consist of quantum assist vector machines, quantum neural networks, and quantum variational algorithms. These algorithms are designed to address precise system mastering tasks on quantum hardware.
Wrap Up
The convergence of quantum computing and device-gaining knowledge has the potential to transform fields that include drug discovery, optimization troubles, economic modelling, and more. Quantum machine learning algorithms have shown promise in solving complex issues beyond the capabilities of classical computers.
Quantum machine learning is a rising area that combines the ideas of quantum computing with system learning algorithms. It holds exquisite promise for future development and has the potential to transform various industries.
READ MORE!!!!!