Self-supervised learning is a branch of deep learning that enables AI fashions to study considerable quantities of unlabeled records. By using the inherent shapes and styles in the information itself, those models can extract meaningful representations and features without relying on explicit human annotations. This ability to examine unlabeled records makes self-supervised getting to know in particular precious in eventualities where labeled datasets are scarce or luxurious to reap.
Introduction
In the arena of artificial intelligence (AI) and device getting to know, self-supervised getting to know has emerged as an effective method that is revolutionizing how AI structures examine and apprehend records. Unlike conventional supervised studying techniques, in which labeled records are required, self-supervised studying leverages unsupervised mastering strategies to train AI models in an autonomous and green manner.
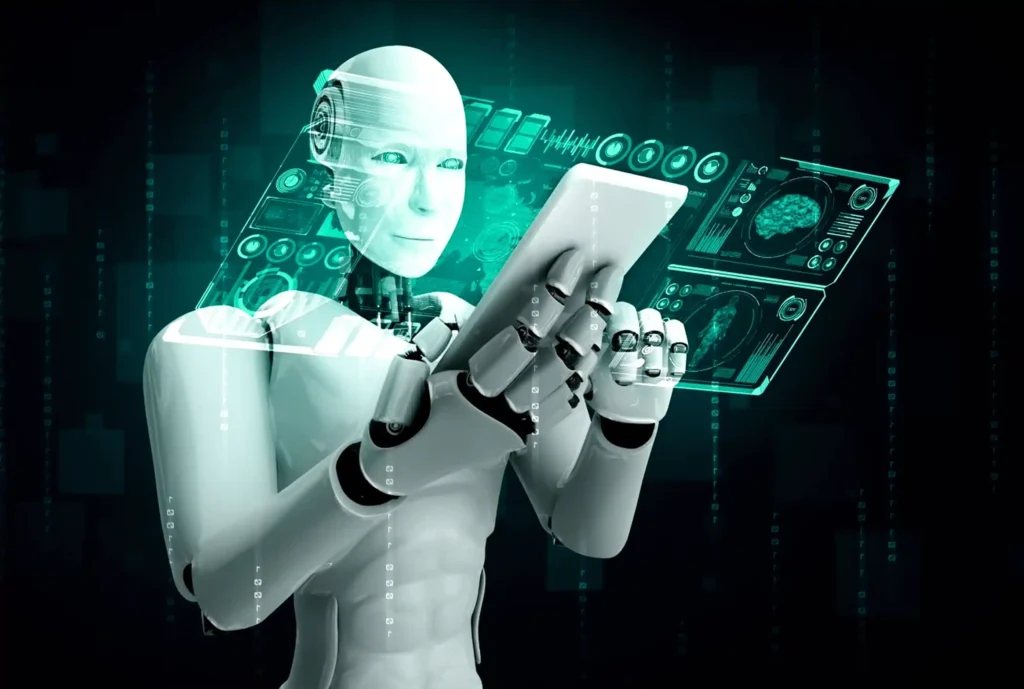
The significance of self-supervised learning lies in its potential to release new possibilities for AI structures. By allowing machines to autonomously extract understanding from uncooked, unannotated facts, self-supervised gaining of knowledge allows for greater scalable and versatile AI applications across diverse domain names, which include laptop vision, herbal language processing, and robotics.
In this segment, we can delve deeper into the principles behind self-supervised learning, explore its key techniques and algorithms, and discuss its implications for the future of artificial intelligence. We can even have a look at some real-global use instances where self-supervised getting to know has established its effectiveness in solving complicated troubles. So, let’s embark on this adventure to find the fascinating global of self-supervised studying in AI.
How Self-Supervised Learning Works: A Step-by-Step Explanation
Self-supervised studying is an effective approach to the subject of artificial intelligence and device mastering that lets machines research unlabeled information. It involves training people to expect positive factors from the facts without explicit labels or annotations. One commonplace approach utilized in self-supervised getting to know is through pretext duties.
Pretext obligations are designed to create a surrogate assignment for the model to solve, which indirectly allows it to learn useful representations of the input records. These responsibilities can range from predicting missing parts of a picture, filling in masked words in a sentence, or maybe predicting the order of shuffled image patches.
The key thing in self-supervised learning is the encoder-decoder structure. The encoder takes in uncooked input information and transforms it into a meaningful, characteristic illustration. The decoder then attempts to reconstruct the authentic input from this selection representation. By forcing the version to encode and decode equal records, it learns to extract excessive-level capabilities that capture vital styles and systems within the statistics.
To train those styles, a contrastive loss feature is commonly employed. This loss feature encourages similar times to have comparable representations while pushing assorted instances apart. It ensures that similar inputs are mapped close together in the function area at the same time as multiple inputs are driven further aside.
Step-by-Step, Self-Supervised Studying Entails:
1. Choosing a pretext undertaking that defines how the model ought to expect certain components of unlabeled information.
2. Designing an encoder-decoder architecture that could transform uncooked input into significant function representations.
3. Training the model to minimize the usage of large amounts of unlabeled records by minimizing a contrastive loss characteristic.
4. Fine-tuning the found-out representations on downstream tasks, inclusive of category or regression.
Self-supervised learning has received enormous interest due to its ability to leverage sizeable quantities of unlabeled statistics available on the net for schooling AI fashions efficaciously. By enabling machines to study unannotated information, self-supervised learning opens up new possibilities for enhancing performance across diverse domains and applications.
The Advantages and Applications of Self-Supervised Learning in AI
Self-supervised learning has emerged as an effective technique within the discipline of synthetic intelligence (AI). Unlike traditional supervised studying techniques that require categorized facts, self-supervised getting to know lets in AI fashions to analyze unlabeled facts. This method gives numerous blessings and has determined applications in various domain names.
One of the key advantages of self-supervised studying is unsupervised characteristic learning. By training AI models on large amounts of unlabeled records, they can routinely study meaningful representations or functions without the need for explicit labels. These found-out features can then be used for downstream tasks, which include class or regression, leading to stepped-forward performance.
Another benefit is record-green schooling. Self-supervised learning enables AI models to leverage substantial quantities of unlabeled facts with no trouble, which is regularly less difficult to achieve in comparison to categorized statistics. This reduces the reliance on expensive and time-consuming labeling processes, making it more cost-effective and scalable.
Transfer-gaining knowledge is another application of self-supervised learning. Pretrained models educated using self-supervision may be used as a starting point for different tasks with constrained, categorized facts. The know-how received at some point in self-supervised training can be transferred to new tasks, improving generalization and lowering the need for considerable project-specific categorized datasets.
Self-supervised learning has shown promising effects in laptop imaginative and prescient duties, which include image reputation, object detection, and photo technology. By leveraging the inherent shape within pix, AI models can study wealthy representations that capture meaningful visual records.
In herbal language processing tasks, self-supervised gaining of knowledge has also been validated. Models that educate the use of self-supervision can analyze contextualized phrase embeddings or language representations that seize semantic relationships between phrases or sentences. This allows higher overall performance in tasks including sentiment analysis, device translation, and textual content summarization.
Self-supervised learning offers several advantages in AI studies and programs. By harnessing unlabeled statistics and leveraging unsupervised feature learning strategies, it opens up new opportunities for extra-efficient training strategies, improved performance, and wider applicability across diverse domain names.
Challenges and Limitations of Self-Supervised Learning
Self-supervised studying is a promising approach to getting to know devices, wherein models are educated to analyze unlabeled information. While this method has proven brilliant capacity, it also comes with a fair percentage of demanding situations and obstacles that want to be addressed.
One of the main challenges in self-supervised studying is curriculum layout. Designing a powerful curriculum entails figuring out the order and problem of training examples supplied to the model. Finding the right balance between smooth and difficult examples can substantially impact the model’s ability to generalize nicely to unseen facts.
Another project lies in poor sampling strategies. Negative samples are important for training self-supervised fashions as they help distinguish between nice and negative times. However, deciding on informative bad samples that closely resemble fantastic times can be difficult, and incorrect sampling techniques may additionally lead to biased or suboptimal fashions.
Evaluation metrics pose yet another assignment in self-supervised learning. Unlike supervised getting-to-know responsibilities, wherein accuracy or precision-bear-in-mind curves are usually used as metrics, comparing self-supervised fashions requires defining suitable assessment criteria particular to each undertaking. Developing reliable assessment metrics is crucial for correctly assessing the model’s overall performance and evaluating exclusive approaches.
It is vital to recognize those demanding situations and work towards addressing them so that you can fully leverage the capacity of self-supervised mastery. Continued studies and innovation in curriculum layout, negative sampling techniques, and evaluation metrics will make contributions in the direction of improving the effectiveness and applicability of this approach in numerous domains of system learning.
Promising Approaches and Cutting-Edge Research in Self-Supervised Learning
Self-supervised gaining knowledge is a hastily evolving field in device-gaining knowledge that has won full-size attention in recent years. It offers promising tactics and cutting-edge research for education models without the need for labeled statistics.
One of the outstanding strategies in self-supervised studying is contrastive predictive coding (CPC). This approach makes a specialty of predicting destiny representations of facts using the temporal shape within it. By educating a model to predict future time steps, CPC permits the model to learn significant representations that seize essential functions of the entered information.
Another thrilling method is BYOL (Bootstrap Your Own Latent), which aims to research effective representations through a manner of online adaptation. BYOL combines online and offline networks, where the web community predicts goal representations from augmented views of input records, while the offline community serves as a goal community for updating weights. This technique has shown promising consequences in numerous domains, which include laptop vision and herbal language processing.
SimCLR (Simple Framework for Contrastive Learning) is another modern-day approach that specializes in maximizing agreement among, in another way, augmented perspectives of an entry pattern. By contrasting high-quality pairs against terrible pairs, SimCLR learns powerful representations that capture high-stage semantic facts from unlabeled statistics. This technique has validated impressive performance across numerous responsibilities, along with photograph type and illustration learning.
These processes in self-supervised studying show how researchers are pushing the boundaries of unsupervised training techniques. They provide thrilling opportunities for education models with restrained labeled information or maybe unlabeled datasets, paving the way for improvements in regions inclusive of transfer learning, semi-supervised learning, and generalization competencies of AI systems.
The Impact of Self-Supervised Learning on the Future of AI
Self-supervised learning is revolutionizing the sphere of synthetic intelligence by allowing machines to clear up complicated international issues without counting on categorized statistics. This modern method has the potential to significantly lessen the time and effort required for manual annotation in AI studies.
AI models have closely relied on large amounts of categorized statistics to examine and make accurate predictions. However, obtaining such records may be an arduous and highly expensive process. Self-supervised learning offers a promising opportunity to leverage unlabeled records to teach fashion.
Through the use of self-supervised mastering techniques, AI models can study the inherent shapes and patterns present in the unlabeled data. They can extract significant representations and construct a comprehensive understanding of the underlying concepts without explicit human annotations.
This paradigm shift has far-achieving implications for diverse domains, which include laptop imaginative and prescient, natural language processing, and robotics. It allows AI systems to generalize more, adapt to new eventualities more efficaciously, and carry out responsibilities with constrained labeled statistics.
Reducing reliance on guide annotation efforts not only accelerates AI research but also makes it more reachable. Researchers can now focus their efforts on designing innovative algorithms instead of spending a lot of time annotating massive datasets.
Self-supervised learning is poised to shape the future of artificial intelligence by permitting machines to examine unlabeled statistics effectively. This advancement has mammoth potential for solving complex real-global issues while lowering dependency on manual annotation efforts in AI studies.
FAQS
Self-supervised learning is a machine learning paradigm where a model learns from the data itself without the need for external labels. The model generates its labels or representations from the input data, enabling it to discover meaningful patterns and relationships.
Self-supervised learning offers advantages such as leveraging vast amounts of unlabeled data, reducing the need for extensive labeled datasets, and enabling models to learn more robust and abstract features, ultimately improving their generalization capabilities.
Self-supervised learning finds applications in various domains, including natural language processing, computer vision, and speech recognition. It can be used for tasks such as image recognition, language understanding, and feature learning, leading to improved performance in these applications.
Conclusion
Accepting the energy of self-supervised learning can unlock new opportunities in AI development. By utilizing this approach, AI systems can examine full-size amounts of unlabelled facts, enabling them to gather understanding and talents without human supervision.
Embracing self-supervised mastering as a fundamental building block in AI development will pave the way for groundbreaking advancements and improvements. As we continue to discover its talents and refine its techniques, we can anticipate a future where AI structures are more clever, adaptable, and successful than ever before.